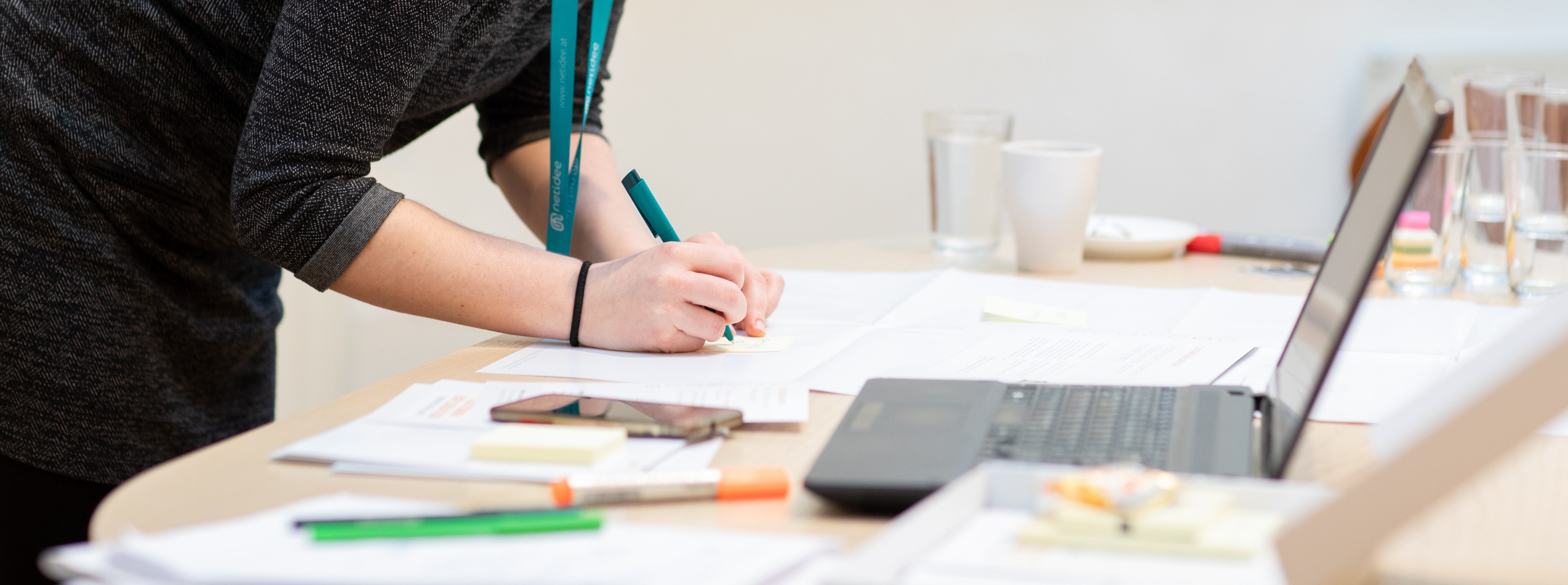
Förderjahr 2023 / Stipendien Call #18 / ProjektID: 6801 / Projekt: Communication and Energy Efficient Edge Artificial Intelligence Framework for Internet of Things
Driven by the rapid advances in Artificial Intelligence (AI) and the Internet of Things (IoT), billions of mobile and IoT devices are connected to the internet, generating huge quantities of data at the network edge. Meanwhile, traditional analytics approaches such as cloud computing and centralized machine learning (ML) are unable to manage these massively distributed heterogeneous data primarily because;
- moving a tremendous amount of data across the network poses severe challenges to network capacity
- cloud-based analytics can result in prohibitively high transmission delays
- transporting data containing private information over the network poses serious concerns for privacy and may not even be possible due to regulations like GDPR.
Accelerated by the success of AI and IoT technologies, there is an urgent need to push AI to the network edge to tap the full potential of big data.
Edge AI
The fusion of artificial intelligence and edge computing has birthed a new paradigm called Edge Intelligence (EI) or Edge AI. Edge AI pushes intelligence closer to where the data is being produced by enabling the deployment of ML algorithms on the edge of the network. Powered by the strengths of AI and edge computing which defines the process of extending computing, storage, and communication resources from a centralized cloud server to the edge of the network with the aim of improving the quality of service (QoS) for mission-critical applications such as connected vehicles, smart cities, and smart environmental monitoring, Edge AI promises to enable new futuristic applications by mitigating the challenges faced by traditional centralized ML.
Edge AI Promises and Challenges
Numerous applications such as smart health, internet of vehicles (IoVs), and smart environmental monitoring (SEM) require performance and privacy assurances, low latency and faster inference, bandwidth and energy efficiency, and scalability. For instance, water quality monitoring (WQM), requires real-time analytics. Failure to promptly detect the presence of hazardous chemicals may have severe health and ecological consequences. Another challenge at the core of these applications is privacy. For example, multiple hospitals that might be interested in collaboratively training a model may not be inclined or even allowed to share their private data. The Edge AI can respond to these low latency and strict privacy requirements due to its geographically distributed nature in close proximity to the source.
However, the part of Edge AI is filled with numerous obstacles, such as i) restrictions regarding privacy and latency render centralized model training methods essentially ineffective, ii) increased energy consumption exacerbated by decentralized model training, data transmission, and storage of big data, and iii) system and data heterogeneity inherent to most IoT applications deteriorate the performance of the system. The gaol is to implement novel and autonomic solutions to the aforementioned challenges.