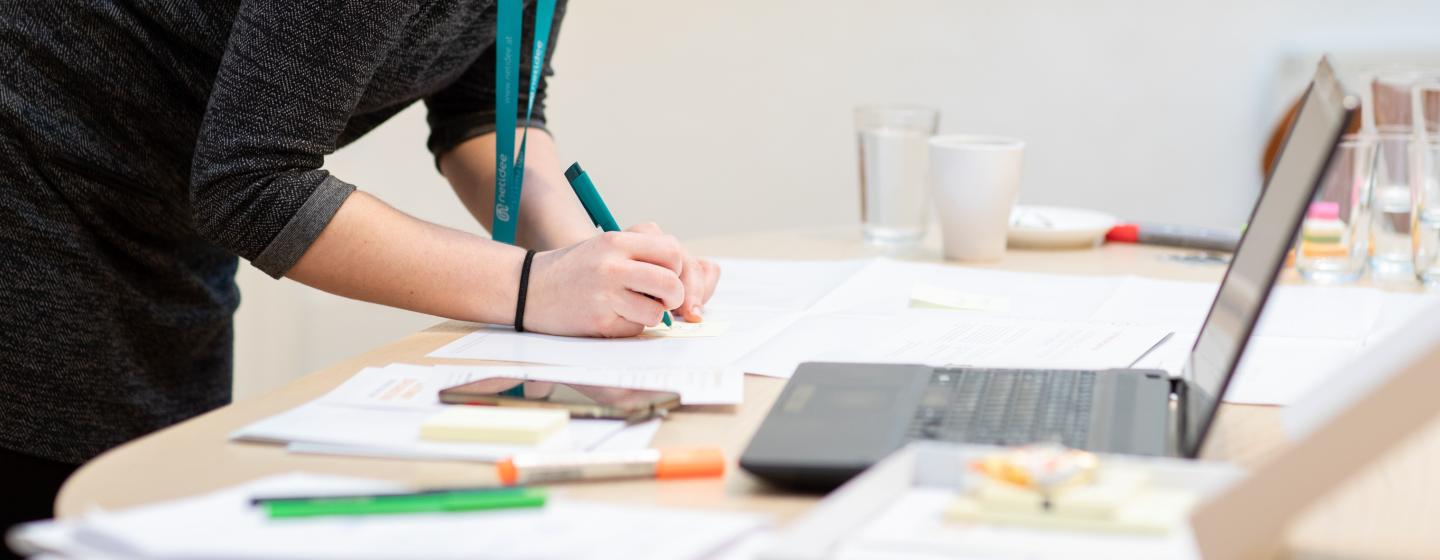
Förderjahr 2021 / Stipendien Call #16 / ProjektID: 5843 / Projekt: Impact of Artificial Intelligence on Women’s Human Rights
Artificial intelligence can be described as a system that has a certain kind of intelligence, a behaviour to analyse its environment and being able to take actions with some degree of autonomy.[1] The learning mechanism in the background of the system can be set up in different ways. The easiest way of learning is the process of trial and error, in which the system learns by memorizing different solutions, which of those to recall for which problem. A more challenging learning method is the so-called generalisation. By doing so, those past experiences are applied to analogous new situations.[2]
If something is biased, it means ‘preferring or disliking someone or something more than someone or something else, in a way that means that they are treated unfairly’ or ‘giving results that are not accurate because the information has not been collected correctly’, as by the Cambridge Dictionary.[3] Artificial intelligence may be biased for two reasons. First, because cognitive biases were either by the designers unknowingly introduced in the model or because a training data set includes those biases. The second possibility of a bias in AI is a lack of complete data, meaning to not be representative.[4] A lot of our bias in AI is a human-made problem, as ‘humans carry (un)conscious biases and behave accordingly’ and by that ‘human biases find their way into the historical data that is used to train algorithms’[5].
An example of a bias in AI concerning gender inequality would be Amazon’s recruiting algorithm that learned by historical data of technical roles being filled by males, that resumes including the word ‘women’ are to be penalized.[6] Detection of those differences can show ‘long-standing stereotyped views and prejudice in society’, which might end up in discrimination.[7] Gender bias in human-made systems is a well-known problem, and there are several examples showing that it may have an adverse effect on women. For example, car seatbelts, headrests and airbags are all designed according to the male’s physique and seat position. This measurement is used as a ‘standard' that does not fit for women’s breasts and pregnant bodies. Resulting, ‘women are 47% more likely to be seriously injured and 17% more likely to die than a man in a similar accident’.[8]
Therefore, it is not surprising that humans’ prejudice against gender is found in artificial intelligence systems. For example, through gender bias in AI, there is a higher error rate in identifying women in computer vision systems, especially when women have darker skin.[9] Gender bias in artificial intelligence thus results in 70% ‘lower quality of service for women and non-binary individuals’.[10] ‘Gender-biased AI not only has immense impacts on peoples but can also contribute to setbacks in gender equality and women’s empowerment’.[11] Classified, there are several biases, which can be distinguished: biases in voice and speech recognition as well as in face recognition, biases in recruiting tools, search engines and in health-related and criminal justice applications.[12]
[1] ACRAI, Artificial intelligence <https://acrai.at/en/home/>.
[2] Copeland, Britannica, Artificial Intelligence <https://britannica.com/technology/artificial-intelligence/Alan-Turing-and-the-beginning-of-AI>.
[3] Cambridge Dictionary, biased <https://dictionary.cambridge.org/de/worterbuch/englisch/biased>.
[4] Kantarci, AI Multiple, Bias in AI: What it is, Types & Examples of Bias & Tools to fix it < https://research.aimultiple.com/ai-bias/>.
[5] Ebert, Mostly AI, Why Bias in AI is a Problem & Why Business Leaders Should Care (Fairness Series Part 1) <https://mostly.ai/2020/05/04/why-bias-in-ai-is-a-problem/>.
[6] Ebert <https://mostly.ai/2020/05/04/why-bias-in-ai-is-a-problem/>.
[7] Gerards, The fundamental rights challenges of algorithms, Netherlands Quarterly of Human Rights (2019) 37/3, 207.
[8] Niethammer, Forbes, AI Bias Could Put Women’s Lives At Risk – A Challenge For Regulators, Forbes <https://forbes.com/sites/carmenniethammer/2020/03/02/ai-bias-could-put-womens-lives-at-riska-challenge-for-regulators/?sh=1c70d229534f>.
[9] Feast, Havard Business Review, 4 Ways to Address Gender Bias in AI, Harvard Business Review <https://hbr.org/2019/11/4-ways-to-address-gender-bias-in-ai>.
[10] Smith/Rustagi, Stanford Social Innovation Review, When Good Algorithms Go Sexist: Why and How to Advance AI Gender Equity <https://ssir.org/articles/entry/when_good_algorithms_go_sexist_why_and_how_to_advance_ai_gender_equity>.
[11] Smith/Rustagi <https://ssir.org/articles/entry/when_good_algorithms_go_sexist_why_and_how_to_advance_ai_gender_equity>.
[12] European Commission, Women in Artificial Intelligence: mitigation the gender bias < https://ec.europa.eu/jrc/communities/en/community/humaint/news/women-artificial-intelligence-mitigating-gender-bias>.