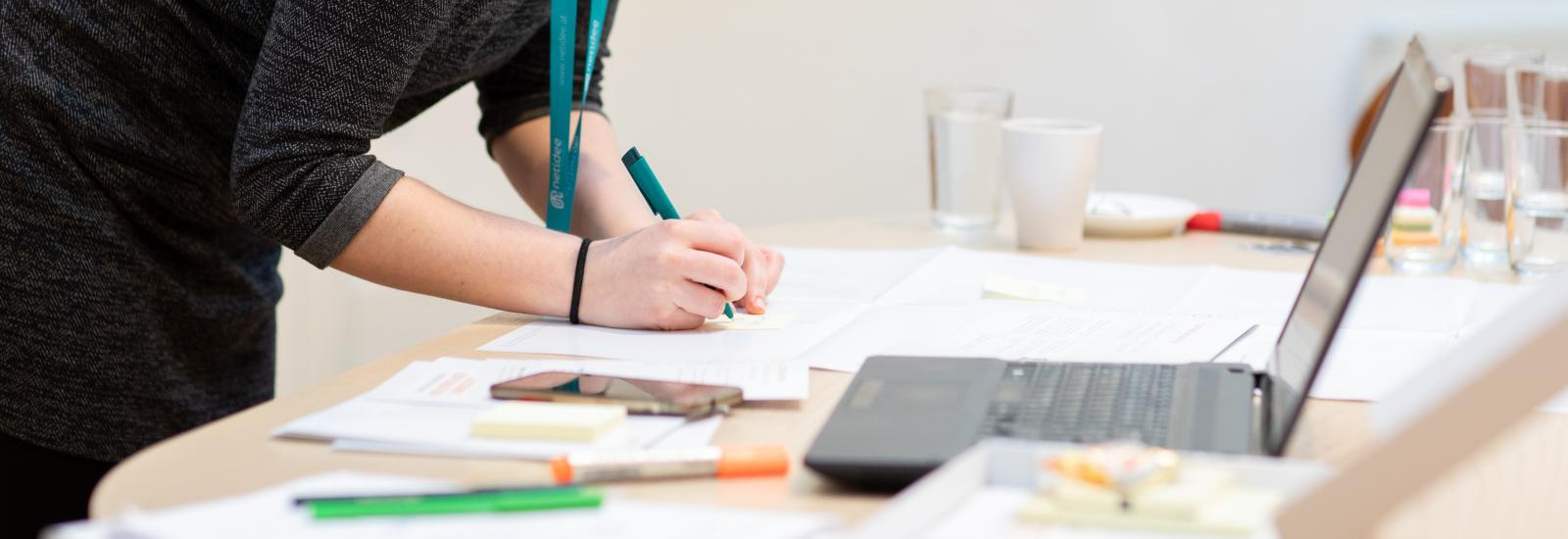
Förderjahr 2021 / Stipendien Call #16 / ProjektID: 5843 / Projekt: Impact of Artificial Intelligence on Women’s Human Rights
While doing research for my diploma thesis, I came across so many examples of gender biases in artificial intelligence, I had not heard about before. That’s why, I would like to give a very short overview of some examples in this blog – to share this information and make it possible, that more people get aware of it! More information can be found in the cited links!
1. Facial Recognition Systems: It was found out, that facial analysis software cannot, or only with a higher error rate, detect dark-skinned faces and faces of women. Error rates for lighter-skinned men have been under 1% compared to results for darker-skinned women with error rates of up to 35%![1]
2. Recruiting Tools: Amazon’s recruiting system was trained to observe patterns in previous resumes and apply them to current applications. However, most people working in US tech companies are men. Using this data, the AI penalized those resumes including the word ‘women’s’ as in ‘women’s chess club captain’ or ‘women’s colleges’. Therefore, the algorithm in job ratings was also based on gender biases.[2]
3. Advertisement: An experiment carried out by Algorithm Watch showed discriminatory practices in job advertisements on the platforms Facebook and Google. Without permission, the platforms, targeted posted ads by Algorithm Watch, which resulted in highly gendered advertisements. In one experiment group, the job for truck drivers has been shown to 4.864 men but only 386 women; the job for childcare workers, contrary, has been shown to 6.456 women but only 258 men.[3]
4. Image Search: A study has been carried out on Google image search results on different occupations, compared to actual statistics on women working in this field. In image searches for CEOs, only 11% were depicted as women, compared to 27% of women working as CEOs in the United States.[4] In a later study, Google image search results have been investigated again. For certain terms such as ‘CEO’ gender fairness can be observed, however, that is not the case in similar search results. Search engines have not been gender-neutral to variants of the original search term, for example, ‘CEO US’ or ‘CEO UK’ instead of ‘CEO’ only. Therefore, gender bias is still evident, in some search engines with variants of the original dataset of occupations.[5]
5. Word Embeddings: Word embeddings represent words or common phrases and serve as a dictionary for computer programs that use word meanings. As an example, for the analogy puzzle, ‘man is king as woman is to x’, embedding vectors find that ‘x=queen’ as being the best answer. The same system solving those word embedding analogies would offensively also refer to a man as a computer programmer as to a woman as a home-maker. ‘Similarly, it outputs that a father is to a doctor as a mother is to a nurse’.[6] A lot more of those examples can be found in the cited article.
6. Credit Scoring Systems: A couple, separately applying for a credit line increase with Apple Card, found the program to be sexist. The couple has been married, and they have been living together for a long time. The woman has had a better credit score and other factors on her side, but her application has been denied. The man had a credit line, which was 20 times higher than the one of his wife.[7]
A different case has been reported in Germany, in which an online purchase on account from a woman has been declined. She did not have any debts and had a good income. Calling customer service on this, she got the reply that the decision was probably made based on her age and gender.[8]
7. Digital Assistants: An investigation was made on voice assistants regarding sexual harassment. For example, Siri’s answer to the statement ‘you’re a bitch’ was ‘I’d blush if I could’. Responses of voice assistants to verbal harassment have shown, that besides Google home, which didn’t understand most of the sexual gestures, the bots mostly evaded harassment, sometimes reacted positively with graciousness or flirtation. There has been barely any response in a way to stop such harassment or to make the inappropriateness clear. To questions of ‘What is rape’ or ‘Is rape okay’ there have been some disturbing answers by not understanding the question or producing internet searches in which one of the top hits was a video titled “When Rape is Okay”. Voice assistants in how they are programmed ‘reinforce stereotypes of unassertive, subservient women in service positions’. The responses given by the voice assistants would give reason to believe that they encourage the idea that silence means ‘yes’ instead of defending healthy communication about consent. [9]
[1] Joy Bualamwini, ‘Artificial Intelligence Has a Problem With Gender and Racial Bias. Here’s How to Solve It’ Time (7 February 2019) <time.com/5520558/artificial-intelligence-racial-gender-bias/>.
[2] Jeffrey Dastin, ‘Amazon scraps secret AI recruiting tool that showed bias against women’ (11 October 2018) <reuters.com/article/us-amazon-com-jobs-automation-insight-idUSKCN1MK08G>.
[3] Nicolas Kayser-Bril, ‘Automated discrimination: Facebook uses gross stereotypes to optimize ad delivery’ (Algorithm Watch, 18 October 2020), algorithmwatch.org/en/automated-discrimination-facebook-google/.
[4] Jennifer Langston, ‘Who’s a CEO? Google image results can shift gender biases’ (9 April 2015), University of Washington News, <washington.edu/news/2015/04/09/whos-a-ceo-google-image-results-can-shift-gender-biases/>
[5] Yunhe Feng and Chirag Shah, ‘Has CEO Gender Bias Really Been Fixed? Adversarial Attacking and Improving Gender Fairness in Image Search’ (2022) Proceedings of the AAAI conference on artificial intelligence, 2f, <yunhefeng.me/material/Bias_in_Image_Search_AAAI22_Feng.pdf>.
[6] Tolga Bolukbasi and others, ‘Man is to Computer Programmer as Woman is to Homemaker?’ 1, 2f.
[7] Neil Vigdor, ‘Apple Card Investigated After Gender Discrimination Complaints: A prominent software developer said on Twitter that the credit card was “sexist” against women applying for credit’ (10 November 2019) <nytimes.com/2019/11/10/business/Apple-credit-card-investigation.html>.
[8] Sarah Michot and others, ‘Algorithmenbasierte Diskriminierung: Warum Antidiskriminierungsgesetze jetzt angepasst werden müssen’ (Algorithm Watch, Digital Autonomy Hub, Februry 2022), <algorithmwatch.org/de/wp-content/uploads/2022/02/DAH_Policy_Brief_5.pdf>.
[9] Leah Fessler, ‘We tested bots like Siri and Alexa to see who would stand up to sexual harassment’ (22 February 2017) <qz.com/911681/we-tested-apples-siri-amazon-echos-alexa-microsofts-cortana-and-googles-google-home-to-see-which-personal-assistant-bots-stand-up-for-themselves-in-the-face-of-sexual-harassment/>.