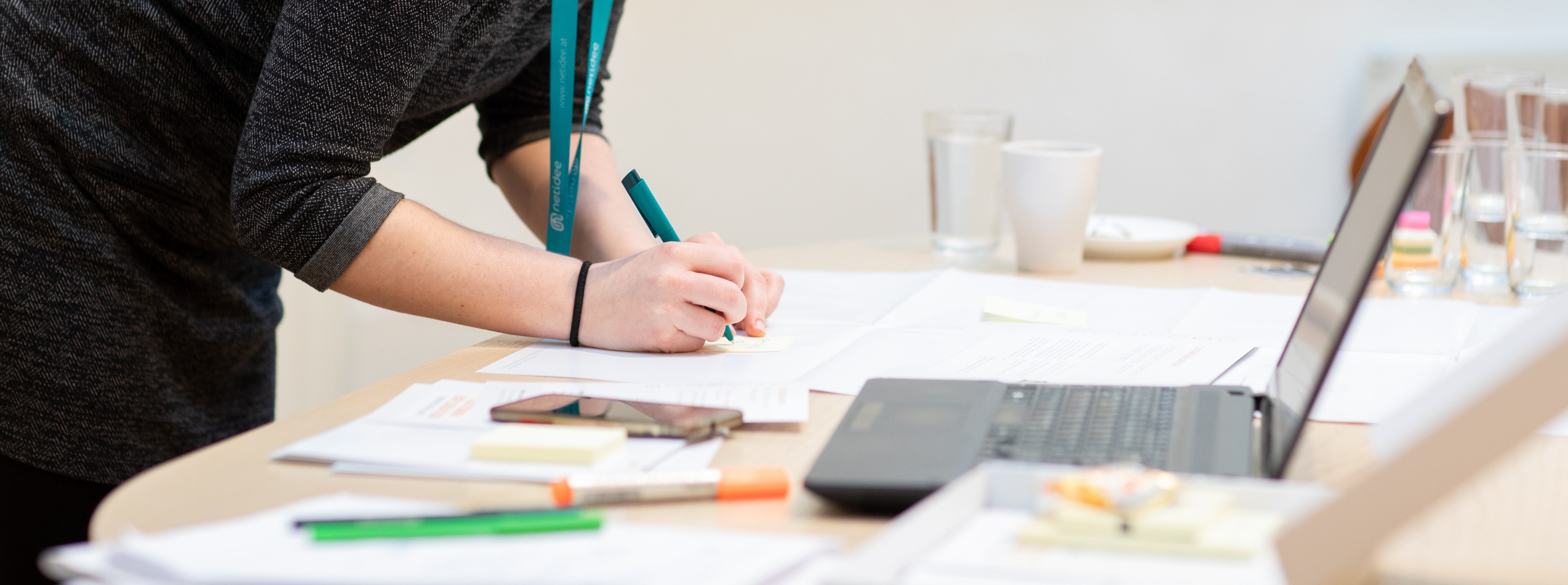
Förderjahr 2018 / Stipendien Call #13 / ProjektID: 3793 / Projekt: Data Management Strategies for Near Real-Time Edge Analytics
This blog post gives an update to the state-of-the-art of edge data management strategies for enabling reliable decision-making for important predictive analytics in the context of storage-limited edge nodes.
Since IoT is highly distributed and decentralized, deployment of edge data management solutions in the proposed EDMFrame employ different areas and cover viewpoints such as data management, data science, and industrial viewpoint.
Data management viewpoint
There are different data management strategies for IoT and edge systems, considering IoT requests offloading [1], IoT resource management [2], and IoT security mechanism [3]. Authors in [4] target the resilience and privacy of sensitive data in delay tolerant networks. Other solutions [5], [6], describe the interplay and communication models for cloud and IoT resources due to growing data streaming and increasing latency issues of smart sensors. However, mentioned solutions do not discuss critical decision-making processes at the edge and focus only on QoS for distributed edge data processing, workload management and ensuring the security of IoT sensitive data rather than considering data reconstruction and storage management. Although some works such as [7] propose various reconstruction methods of incomplete datasets, they do not distinguish recovery of various gaps, despite diverse data characteristics. For timely and accurate data recovery in modern IoT systems, it is necessary to combine different recovery techniques, even within the same datasets.
Data science viewpoint
Methods like ARIMA and ETS are very suitable for near real-time decisions in IoT since they do not require much user interaction. Further, despite scenarios where data generation is triggered by certain events, here I focus on regularly time-stamped measurements. However, EDMFrame is designed in a generic way, such that depending on the application context and sensor data characteristics, different methods can be utilized for both data recovery mechanisms and adaptive edge storage management and can be adapted to different application-areas and their requirements.
Existing industrial frameworks
Collection and data analysis at the edge is the basis of industrial cloud platforms such as AWS IoT Greengrass, which performs data storage on the cloud, rather than on the edge; Azure IoT Edge employs containers to package modules and custom logic at the edge. AWS IoT Analytics offers remote device management, optimized IoT data storage, and time-series analytics, enabling end-to-end workflow automation for large amounts of data and connecting IoT devices with cloud applications. Several frameworks for IoT data processing have been proposed such as Eclipse Kura, Node-RED and Flogo. Eclipse Kura represents a reference IoT Edge framework for building IoT gateways, incorporating networking protocols, and data services, and allowing connectivity of IoT devices to their cloud platform. Most of these approaches focus on integrating heterogeneous IoT devices and fully managed workload services instead of edge data management on limited storage and adaptive data recovery with multiple techniques. Complementary to these works, EDMFrame is designed as a service built on top of these or similar IoT data processing services, to enhance the data recovery and analytics features they offer.
Storage management viewpoint
The problem of reducing data transmission at the edge nodes, such as micro data centers, has been discussed by several works. In [8], a solution for network-edge data reduction for IoT devices is presented, without considering latency requirements of IoT applications and improvement of data quality by using different forecasting techniques. Paper [9] proposes a dynamic compression-based technique for sensor data. Works like [10], focus on data storage structure, memory allocation strategy, and data compression to improve storage capacity. These challenges have been considered from traditional IoT and cloud perspectives but cannot be used due to edge limited storage.
Furthermore, for future IoT services, it is crucial to make fast and approximate decisions as in smart cities requiring distributed ML at the edge, e.g., in the case of consistent ML models that must be updated when data streams evolve over time, posing critical issues to observe correct data at the right time. ML can be employed to analyze data to help improve decisions. Since ML relies on the training process that trains the model, there is a constant need for model retraining as more data becomes available. Hence, one of the goals is to explore using EDMFrame also for reliable distributed ML at the edge.
References:
[1] Al-Khafajiy, Mohammed, et al. "IoT-Fog Optimal Workload via Fog Offloading." 2018 IEEE/ACM International Conference on Utility and Cloud Computing Companion (UCC Companion). IEEE, 2018. [2] Oueida, Soraia, et al. "An edge computing based smart healthcare framework for resource management." Sensors 18.12 (2018): 4307. [3] Abbas, Nadeem, et al. "A mechanism for securing IoT-enabled applications at the fog layer." Journal of Sensor and Actuator Networks 8.1 (2019): 16. [4] Montella, Raffaele, Mario Ruggieri, and Sokol Kosta. "A fast, secure, reliable, and resilient data transfer framework for pervasive IoT applications." IEEE INFOCOM 2018-IEEE Conference on Computer Communications Workshops (INFOCOM WKSHPS). IEEE, 2018. [5] Van den Abeele, Floris, et al. "Integration of heterogeneous devices and communication models via the cloud in the constrained internet of things." International Journal of Distributed Sensor Networks 11.10 (2015): 683425. [6] Maamar, Zakaria, et al. "Cloud vs edge: Who serves the Internet‐of‐Things better?." Internet Technology Letters 1.5 (2018): e66. [7] Wellenzohn, Kevin, et al. "Continuous imputation of missing values in streams of pattern-determining time series." (2017): 330-341. [8] Papageorgiou, Apostolos, Bin Cheng, and Ernö Kovacs. "Real-time data reduction at the network edge of Internet-of-Things systems." 2015 11th International Conference on Network and Service Management (CNSM). IEEE, 2015. [9] Ukil, Arijit, Soma Bandyopadhyay, and Arpan Pal. "Iot data compression: Sensor-agnostic approach." 2015 Data Compression Conference. IEEE, 2015. [10] Yan, Qi, and Yong-Yan Wang. "A kind of efficient data archiving method for historical sensor data." 2016 3rd International Conference on Information Science and Control Engineering (ICISCE). IEEE, 2016.