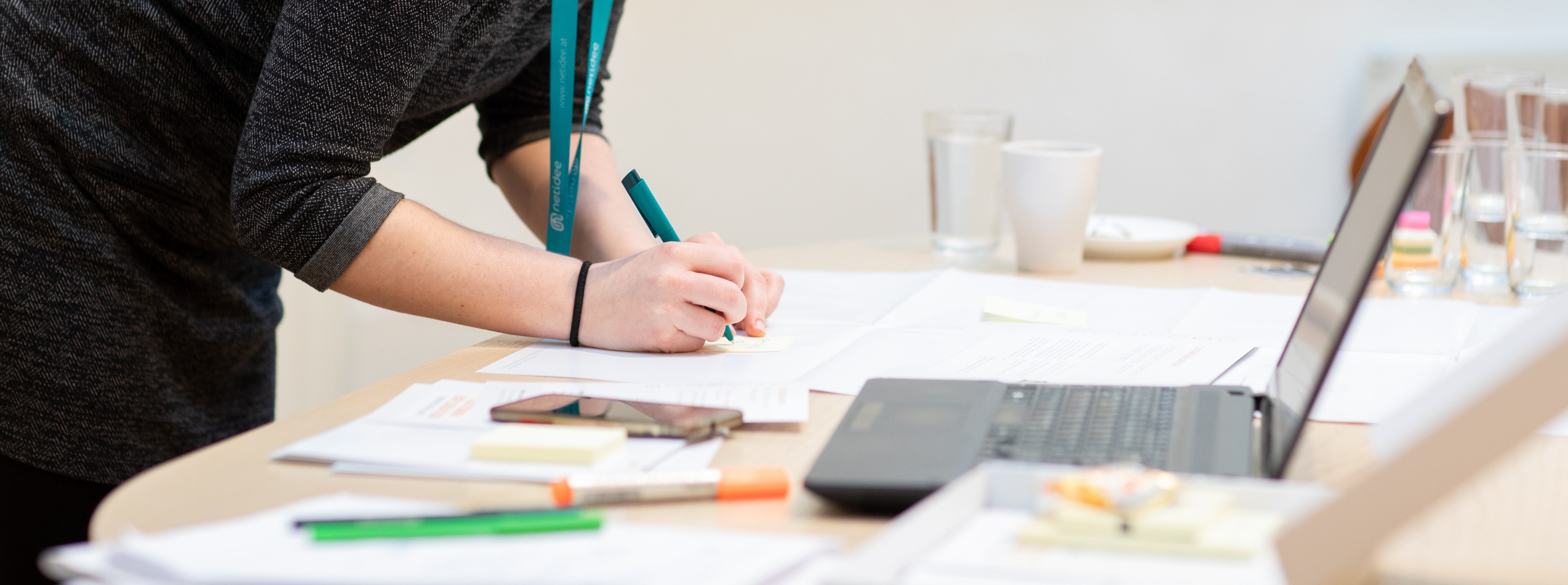
Förderjahr 2018 / Stipendien Call #13 / ProjektID: 3793 / Projekt: Data Management Strategies for Near Real-Time Edge Analytics
Turning opportunities and insights from near real-time sensor data analysis into improved decision-making processes can make IoT systems “intuitive”, leading to needed transformation from reactive to proactive systems.
Edge analytics is a promising solution to meet new strict latency and accuracy requirements from modern IoT systems. These systems, however, should work intuitively and make timely decisions as human bodies but based on sensor data. In this analogy, a human body makes immediate decisions considering current observations and available information, and often relies on intuition.
Modern systems should take proactive decisions
Modern systems are not able to store and analyze all historical data before making near real-time decisions. Therefore, the transition from mathematical paradigm and exact data analysis to statistical paradigm and approaches that incorporate data science methods represent the key building block of this work. Driven by data trends, probability, and approximate data analysis, modern IoT systems should take proactive decisions before the system enters in undesired states asking for appropriate subsequent actions.
Proactivity – the key characteristic of future IoT systems
Our strategies aim to bridge this gap, enabling IoT systems to be more intuitive, where data collected from sensors can be timely analyzed and in which results can timely trigger actuators or send safety notifications for system adaptations to assist systems and actuators to keep overall system performance above a specified threshold.
Data locality and their quality are becoming more and more critical for decision-making in proactive systems. With our proposed strategies, future proactive IoT systems will be able to produce more accurate and timely decisions, e.g., for improving air quality or energy efficiency in smart homes, or in the smart healthcare systems monitoring patients’ vital signs and helping doctors and patients in treating different diseases, as in case of increased sugar level or blood pressure to take medications before something bad happens.
Who can benefit?
System integrators and application developers have important tasks to (i) combine a set of different proposed system subcomponents, (ii) implement strategies along the edge-cloud pipeline and create routines to specify how particular data will be processed among different environments as well as with other applications.
The work described in this thesis can be summarized in three keywords:
- Intuitive decision-making: principles and strategies are made for systems that should work intuitively, like the human body, but based on observed sensor data;
- Data science methods: the proposed strategies rely on approaches different than traditional exact data analysis and include novel data reduction techniques, predictive analytics, and statistical modeling;
- Lifechanging applications: the proposed generic mechanisms can be applied to real-world systems such as smart healthcare, or smart homes, improving data quality and decision-making processes.
Predictive analytics has a huge potential to revolutionize critical and proactive IoT applications, such as accurate diagnosis of patients in smart healthcare, maintenance services and failures prevention in smart manufacturing and building systems. Many works discuss IoT sensor data reduction and dynamic compression techniques, focusing mostly on network optimizations. Still, there is a lack of solutions for accurate predictive analytics while dealing with incomplete data and limited edge storage capabilities. Traditional approaches for IoT and cloud data management address the challenges related to incomplete datasets and storage limitations without considering the impact of data quality and data resilience on decision-making processes, which is of paramount importance to ensure efficient and accurate analytics in IoT systems.
Many existing data management frameworks neglect proactive behavior that represents one of the key elements for future trends in managing IoT systems in edge environments, which will be further addressed in the following months in our research activities of the proposed EDMFrame. Stay tuned!